Research Workshop of the Israel Science Foundation
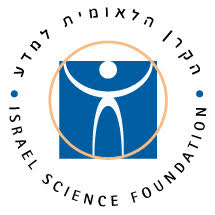
Planning and Learning
The 5th workshop on planning and learning aims to provide a stimulating forum for researchers from both the learning community and the planning community to discuss recent advances, and potential developments on these exciting topics at the intersection of learning and planning.
Proceedings
The full PAL proceedings are available as a pdf file.
Schedule
The workshop will be held on June 8, 2015 in Room D.
13:50-13:55 | Opening Remarks |
13:55-14:45 |
Invited Talk: Joelle Pineau Learning Socially Adaptive Path Planning Strategies |
14:45-16:20 | Paper Presentations |
Kamil Ciosek, David Silver Value iteration with options and state aggregation |
|
David Tolpin, Brooks Paige, Jan Willem van de Meent, Frank Wood Path finding under uncertainty through probabilistic inference |
|
15:25-16:00 | Coffee Break |
Anders Jonsson, Damir Lotinac Automatic generation of HTNs from PDDL |
|
16:20-16:40 | Mark Roberts: IPPC 2014 Learning Track Results. |
16:40-17:30 |
Invited Talk: Wee Sun Lee Maximum Likelihood Determinization |
Accepted Papers
- Kamil Ciosek, David Silver Value iteration with options and state aggregation
- David Tolpin, Brooks Paige, Jan Willem van de Meent, Frank Wood Path finding under uncertainty through probabilistic inference
- Anders Jonsson, Damir Lotinac Automatic generation of HTNs from PDDL
Invited Talks
- Speaker: Joelle Pineau
- Title: Learning Socially Adaptive Path Planning Strategies
- Abstract:
A key skill for mobile robots is the ability to navigate efficiently through their environment. In the case of social or assistive robots, this involves planning paths through human crowds. Typical performance criteria, such as reaching the goal using the shortest path, are not appropriate in such environments, where it is more important for the robot to move in a socially acceptable manner. In this talk I will describe recent methods based on imitation and reinforcement learning which we have developed to allow robots to achieve socially adaptive path planning in human environments. Performance of these methods will be illustrated using experiments with a smart power wheelchair robot called the SmartWheeler. - Bio:
Joelle Pineau is an Associate Professor at the School of Computer Science at McGill University, co-director of the Reasoning and Learning Lab, and member of the Centre for Intelligent Machines (CIM). Her research focuses on developing new models and algorithms for learning and decision-making in partially observable stochastic domains, and applying these models and algorithms to complex problems in robotics and health-care. She is on the editorial board of the Journal of Artificial Intelligence Research and the Journal of Machine Learning Research, and served as the program chair (2012) and general chair (2015) for the International Conference on Machine Learning.
- Speaker: Joelle Pineau
- Speaker: Wee Sun Lee
- Title:Maximum Likelihood Determinization
- Abstract:
Consider a series of problems that combine planning and learning in increasing order of complexity: active learning, informative path planning, and reinforcement learning. In active learning, an agent is interested in identifying a hypothesis with the minimum number of queries to an oracle (usually a human). In informative path planning, an agent is still interested in identifying a hypothesis but needs to plan a path in order to reach a sequence of destinations where information is available. In reinforcement learning, the agent has to identify a Markov decision process while acting in an environment. We consider an adaptive Bayesian setting, and formulate the problem as that of minimizing the expected cost required for identifying a hypothesis or model. We show that one unifying idea, maximum likelihood determinization, can substantially reduce the computational cost of approximating the solutions of these problems. In a nutshell, maximum likelihood determinization is a recursive method for solving these problems, where in each recursive step, we assume the most likely outcome for each observation and solve a non-adaptive planning problem to remove half of the remaining hypotheses. Applied to Bayesian reinforcement learning, we reduce the search space of a look-ahead search by an exponential factor by removing the dependence on the observations, at the cost of a logarithmic approximation factor. For adaptive informative path planning in a metric space, we obtain a polynomial time algorithm with a polylog approximation factor. For Bayesian active learning, the idea results in the commonly used method of selecting the least confident example to query, with a polylog approximation factor. - Bio:
Wee Sun Lee is an Associate Professor in the Department of Computer Science, National University of Singapore. He obtained his B.Eng from the University of Queensland in 1992 and his Ph.D. from the Australian National University in 1996. He has been a research fellow at the Australian Defence Force Academy, a fellow of the Singapore-MIT Alliance, and a visiting scientist at MIT. His research interests include machine learning and planning under uncertainty. His teams won first place in the POMDP track of the ICAPS International Probabilistic Planning Competition 2011 and in 2014. He has been an area chair for the Neural Information Processing Systems conference and a program chair as well as conference chair for the Asian Conference on Machine Learning.
- Speaker: Wee Sun Lee
Call for Papers
Learning and planning are two distinct capabilities required for an intelligent agent, but they are connected in many ways and it is often beneficial to consider them together.
Learning allows planning to be more easily applied to new domains by serving as a means for automatically acquiring knowledge required in planning. In traditional planning methods, knowledge about the models and the search guidance are often manually specified, and can be very difficult or time-consuming to obtain. Many recent works have addressed the knowledge acquisition bottleneck by learning models for planning and learning heuristics to guide planning.
The techniques in planning can be used for solving learning problems. For example, Bayesian reinforcement learning for MDPs can be formulated as a Partially Obserable Markov Decision Process (POMDP), and thus can be solved using a POMDP solution algorithm. The problem of active learning can be formulated as a planning problem too.
Some existing learning or planning algorithms, when viewed from the right perspective, also show interesting connections between learning and planning. For example, adaptive Monte Carlo planning can be seen as online learning of search guidance.
This workshop aims to provide a stimulating forum for researchers from both the learning community and the planning community to discuss recent advances, and potential developments on these exciting topics at the intersection of learning and planning. This workshop is the continuation of the lineage of four workshops on planning and learning in 2007, 2009, 2011, 2013.
Submissions are invited for topics on, but not limited to:
- Multi-agent planning and learning
- Robust planning in uncertain (learned) models
- Adaptive Monte Carlo planning
- Learning search heuristics for planner guidance
- Model-based reinforcement learning
- Bayesian reinforcement learning
- Model representations for learning and planning
- Theoretical aspects of planning and learning
- Learning and planning competition
- Applications of planning and learning
Important Dates
Submission Deadline: February 20, 2015.
Notifications and Technical Program: March 20, 2015.
Workshop Date: June 8th, 2015
Submission Procedure
Paper should be submitted via the workshop EasyChair web site https://easychair.org/conferences/?conf=icapswpal2015.
Paper submission is in PDF only. Please format submissions in AAAI style. Refer to the author instructions on the AAAI web site for detailed formatting instructions and LaTeX style files (http://www.aaai.org/Publications/Author/author.php). Final papers will be in the same format, keep them to at most 8+1 pages long (meaning 8 pages plus 1 extra page containing only references). Please note that all submitted papers will be peer-reviewed, and that low-quality or off-topic papers will not be accepted. Also note that all workshop participants must register for the main ICAPS 2015 conference and that at least one author of each accepted paper must attend the workshop.
Organizing Committee
Alan Fern
School of Electrical Engineering and Computer Science
Oregon State University
Corvallis OR 97331 USA
Hanna Kurniawati
School of Information Technology and Electrical Engineering
The University of Queensland (St. Lucia Campus)
Brisbane, QLD, 4072, Australia
Scott Sanner
NICTA & ANU
Canberra, Australia
Nan Ye
Department of Computer Science
National University of Singapore
Singapore 117417